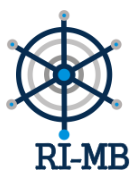
Por favor, use este identificador para citar o enlazar este ítem:
https://www.repositorio.mar.mil.br/handle/ripcmb/846372
Título : | Robust machine learning for computer vision in naval application |
Autor : | Rangel, Gabriel Custódio |
metadata.dc.contributor.advisor: | Eckstrand, Eric C. |
Palabras clave : | Machine learning Computer vision Neural networks |
Áreas de conocimiento de la DGPM: | Engenharia de produção aplicada à pesquisa operacional e gestão da inovação |
Fecha de publicación : | 2023 |
Editorial : | Naval Postgraduate School |
Resumen : | This thesis proposes the development of a resilient machine learning algorithm that can classify naval images for surveillance, search, and detection operations in vast coastal areas. However, real-world datasets may be affected by label noise introduced either through random inaccuracies or deliberate adversarial attacks, both of which can negatively impact the accuracy of machine learning models. Our innovative approach employs Rockafellian Risk Minimization (RRM) to combat label noise contamination. Unlike existing methodologies reliant on extensively cleaned datasets, our two-step process involves adjusting neural network weights and manipulating data point nominal probabilities to isolate potential data corruption effectively. This technique reduces the dependency on meticulous data cleaning, thereby promoting more efficient and timeeffective data processing. To validate the efficacy and reliability of the proposed model, we apply RRM in several parameter configurations to naval environment datasets and assess its classification accuracy against traditional methods. By leveraging the proposed model, we aim to bolster the robustness of ship detection models, paving the way for a novel, reliable tool that could improve automated maritime surveillance systems. |
Access: | Acceso abierto |
URI : | https://www.repositorio.mar.mil.br/handle/ripcmb/846372 |
Type: | Tesis de maestría |
Aparece en las colecciones: | Engenharia Naval: Coleção de Dissertações |
Ficheros en este ítem:
Fichero | Descripción | Tamaño | Formato | |
---|---|---|---|---|
Dissertacao- GabrielCustodioRangel.pdf | 2,56 MB | Adobe PDF | Visualizar/Abrir |
Los ítems de DSpace están protegidos por copyright, con todos los derechos reservados, a menos que se indique lo contrario.